Found 28 talks width keyword Machine learning
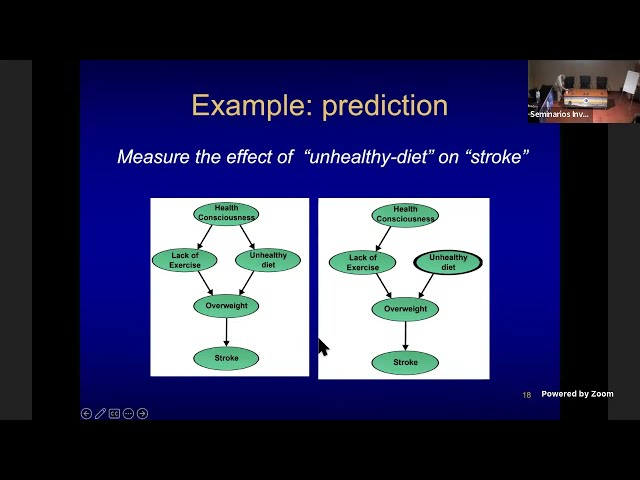
Abstract
Current intelligent systems mainly make "predictions". That is, given an input, they estimate the most probable value of the output. These systems have many limitations, they can be easily confused when presented with a different case from their training set and they cannot explain how they arrive to a certain result. Causal models are an alternative to extend the capabilities of current systems; explain the reasons for certain decisions, predict the effect of interventions and imagine alternative situations. In this talk, I will present an introduction to causal models, in particular to causal graphical models. We will see how we can make inferences based on these models: predictions and counterfactuals; as well as learning causal models from data. I will illustrate the application of causal models in various domains: estimation of effective connectivity in the brain, causal modeling of COVID-19, and incorporating causal models in reinforcement learning and its application in robotics. Finally, I will discuss some potential applications of causal modeling in astrophysics.
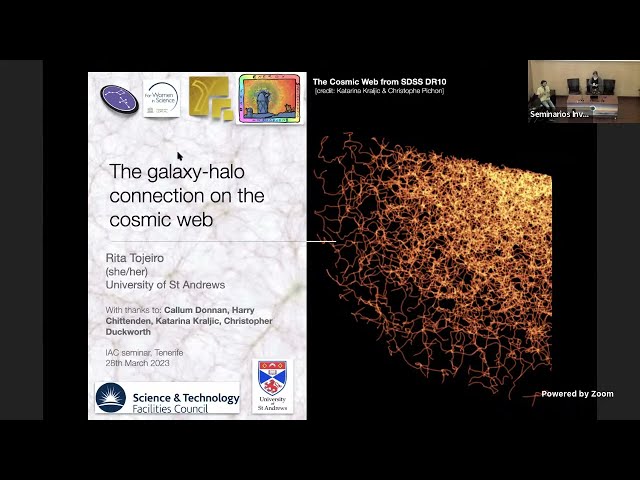
Abstract
Galaxies and the dark matter halos in which they reside are intrinsically connected. That relationship holds information about key processes in galaxy and structure formation. In this talk, I will consider how the galaxy-halo connection depends on position within the cosmic web - the familiar decomposition of large-scale structure in filaments, knots and voids. Simulations demonstrate the various ways in which the cosmic web modulates the growth and dynamics of halos. The extent to which the cosmic web impacts on galaxies is more difficult to establish. For example, galaxies might be sensitive only to the evolution of the host halo, in which case any effect of the cosmic web on galaxies is secondary, and can be inferred from the halo's history. There is evidence, however - from simulations and observations - that the cosmic web also impacts on the evolution of galaxies via the effect it has on the broader gas ecosystem in which they are embedded, as well as through "pre-processing" effects on group scale. So, how should we think of the cosmic web in its role as a transformative agent of galaxies? And what physical processes can we convincingly constrain from observations and simulations? In this talk I highlight recent work that addresses these questions.
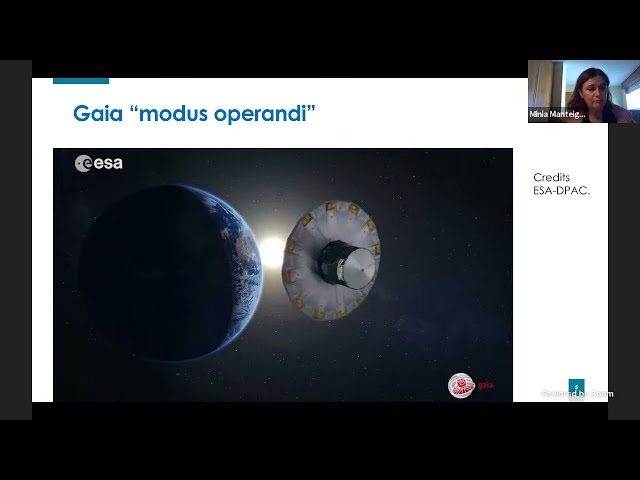
Abstract
After placing the Gaia mission in the context of current astrophysical research, the astrometric, photometric, and spectroscopic data provided by the satellite, as presented in its third data archive, DR3, will be reviewed. Gaia third archive contains, in addition to the measurements from the satellite's instruments, an extensive set of astrophysical parameters derived by the DPAC data processing consortium, for stars, unresolved galaxies, and solar system objects. These parameters correspond to about 1.8 billion sources repeatedly observed by the satellite during the first 34 months of operation. In the case of the Milky Way stars, DR3 contains information for about 1% of its stars, which has made it possible, for the first time, to study the dynamics of the disk and the halo, and to reconstruct the tumultuous evolutionary history of our galaxy, which is etched in its halo. Studying the integrals of the motion and the orbital actions, some 15 episodes of accretion of other galaxies by our galaxy have been identified, which shows that our Galaxy is the result of billions of years of "galactic canibalism". Accretion phenomena and tidal current trails are frequently observed in other galaxies and show that our Universe evolves through a hierarchical formation of galaxies.
-------------------------------------------------
Meeting will be held online
Youtube: https://youtu.be/ns78Go3FM7I
Zoom: https://rediris.zoom.us/j/87455295473?pwd=dnd2SkppQ1F2UlN2K0FLbmlLZEFmZz09
Meeting ID: 874 5529 5473
Passcode: 941406
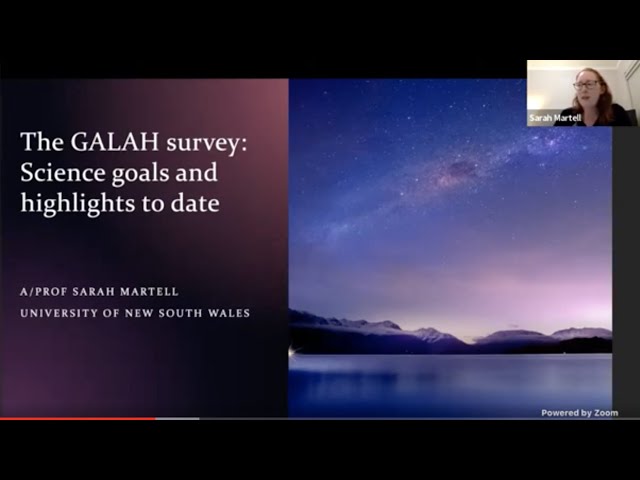
Abstract
The field of Galactic archaeology has been very active in recent years, with a major influx of data from the Gaia satellite and large spectroscopic surveys. The major science questions in the field include Galactic structure and dynamics, the accretion history of the Milky Way, chemical tagging, and age-abundance relations. I will give an overview of GALAH as a large spectroscopic survey, and describe how it is complementary to other ongoing and future survey projects. I will also discuss recent science highlights from the GALAH team and compelling questions for future work.
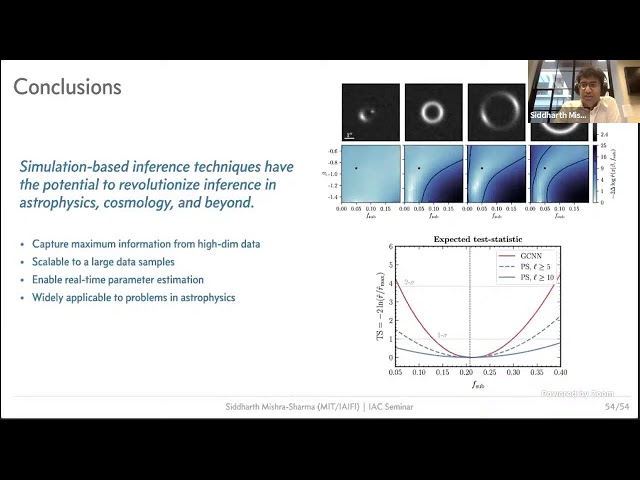
Abstract
The next decade will see a deluge of new cosmological data that will enable us to accurately map out the distribution of matter in the local Universe, image billions of stars and galaxies to unprecedented precision, and create high-resolution maps of the Milky Way. Signatures of new physics as well as astrophysical processes of interest may be hiding in these observations, offering significant discovery potential. At the same time, the complexity of astrophysical data provides significant challenges to carrying out these searches using conventional methods. I will describe how overcoming these issues will require a qualitative shift in how we approach modeling and inference in cosmology, bringing together several recent advances in machine learning and simulation-based (or likelihood-free) inference. I will ground the talk through examples of proposed analyses that use machine learning-enabled simulation-based inference with an aim to uncover the identity of dark matter, while at the same time emphasizing the generality of these techniques to a broad range of problems in astrophysics, cosmology, and beyond.
https://rediris.zoom.us/j/83193959785?pwd=TExXSDJ6UDg5a24yWDM1TnlOWkNTZz09
Meeting ID: 831 9395 9785
Passcode: 343950O
YouTube: https://youtu.be/1Nkzn-cGaIo
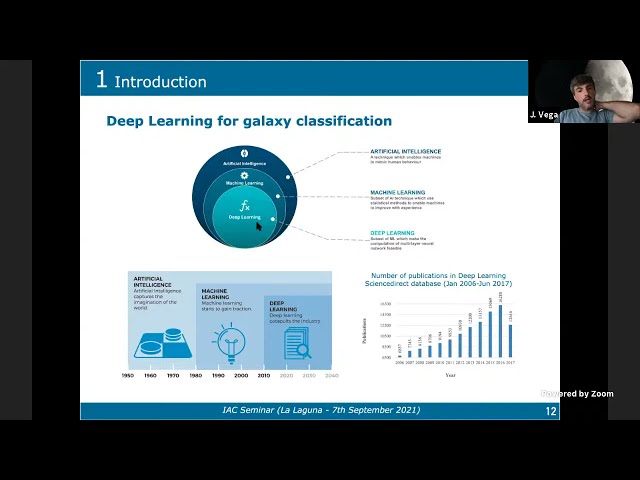
Abstract
Galaxy morphologies are one of the key diagnostics of galaxy evolutionary tracks, but visual classifications are extremely time-consuming. The sheer size of Big Data surveys, containing millions of galaxies, make this approach completely impractical. Deep Learning (DL) algorithms, where no image pre-processing is required, have already come to the rescue for image analysis of large data surveys. In this seminar, I will present the largest multi-band catalog of automated galaxy morphologies to date containing morphological classifications of ∼27 million galaxies from the Dark Energy Survey. The classification separates: (a) early-type galaxies (ETGs) from late-types (LTGs); and (b) face-on galaxies from edge-on. These classifications have been obtained using a supervised DL algorithm. Our Convolutional Neural Networks (CNNs) are trained on a small subset of DES objects with previously known classifications, but hese typically have mr < 17.7 mag. We overcome the lack ofa training sample by modeling fainter objects up to mr < 21.5 mag, i.e., by simulating what thebrighter objects with well-determined classifications would look like if they were at higher redshifts.The CNNs reach a 97% accuracy to mr < 21.5 on their training sets, suggesting that they are ableto recover features more accurately than the human eye. We obtain secure classifications for 87%and 73% of the catalog for the ETG vs. LTG and edge-on vs. face-on models, respectively.
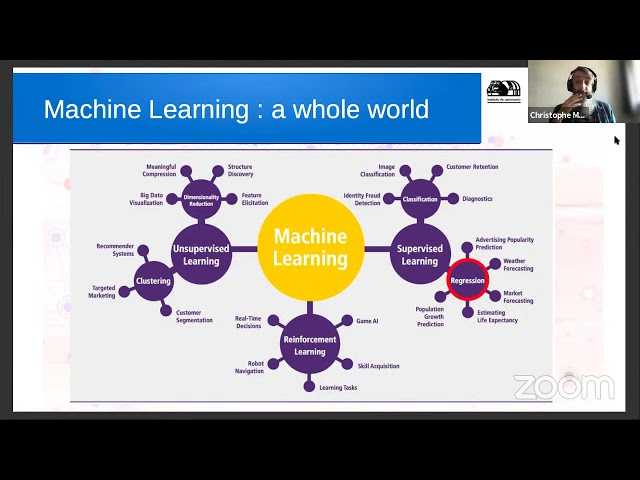
Abstract
Artificial intelligence techniques are increasingly used in our daily lives. They also play an important role in science, including astrophysics. I am particularly interested in the use of machine learning regressors. I will present an overview of the current situation and some recent uses of these methods in the study of planetary nebulae or HII regions.
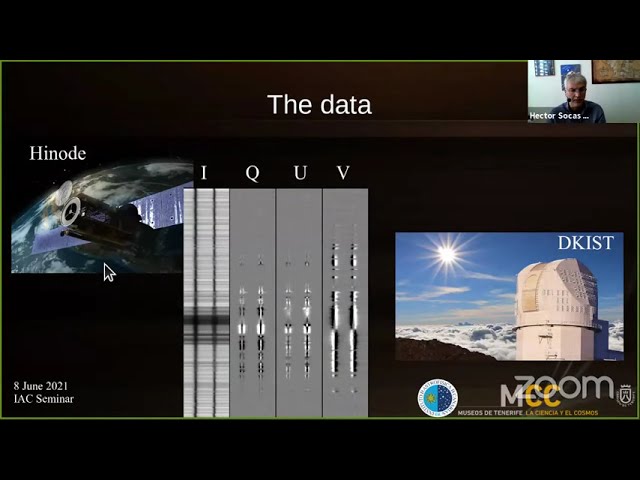
Abstract
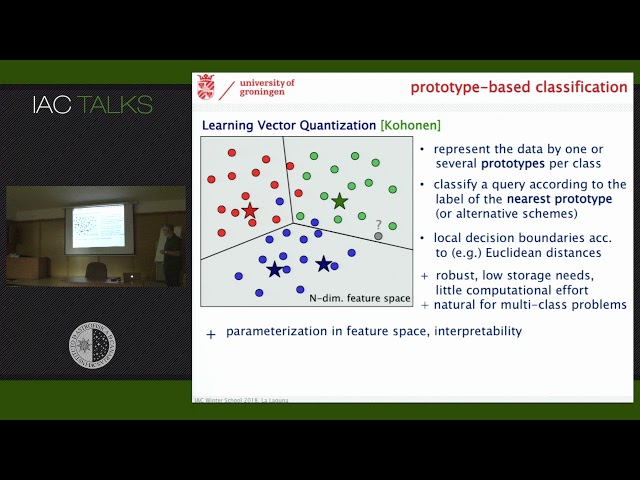
Abstract
Series: XXX Canary Islands Winter School of Astrophysics: Big Data in Astronomy
Topic: Supervised learning: classification and regression
Lecture 4
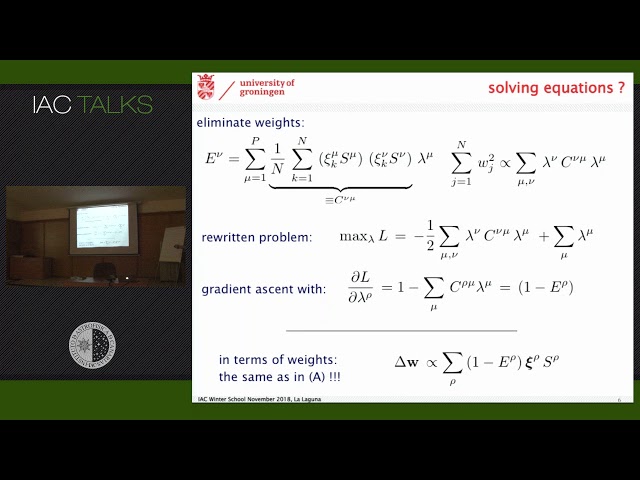
Abstract
Series: XXX Canary Islands Winter School of Astrophysics: Big Data in Astronomy
Topic: Supervised learning: classification and regression
Lecture 3
« Newer 1 | 2 | 3 Older » Last >>
Próximas charlas
- From astronomy to ophthalmology: Adaptive Optics in the eyeProf. Susana MarcosMonday February 24, 2025 - 10:30 GMT (Aula)
- HI content at cosmic noon: a millimeter-wavelength perspectiveDr. Hugo MessiasThursday February 27, 2025 - 10:30 GMT (Aula)